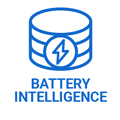
Cambridge EnerTech’s
Battery Intelligence
How Smart Analysis of Your Battery Data Can Drive On-Time New Vehicle Launches, Optimal Performance, and Increased Margins
21-22 June 2023
As the battery market rapidly increases so does the need to optimize lifetime performance. For OEMs, battery pack manufacturers, electric fleet managers, and Electric Vehicle (EV), the key to unlocking battery life lies in the data. Core potential of
battery data when utilizing machine learning and data analytics methods can accurately determine, predict, and improve battery life. To achieve high battery efficiency and operational reliability, predictive intelligence, and data analytics will play
key roles as artificial intelligence becomes more disruptive in the battery technology space. The Battery Intelligence conference will bring thought leaders from industry and academia to discuss how organizations can use battery intelligence to improve
battery life significantly and continuously.