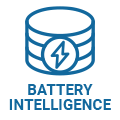
Cambridge EnerTech’s
Battery Intelligence
Using Machine Learning and Artificial Intelligence to Optimise Battery Development from Materials to Manufacturing
15-16 MAY 2024
As the battery market undergoes rapid expansion, the need to optimise the longevity of batteries becomes increasingly evident. For OEMs, battery pack manufacturers, and electric fleet managers, the key to unlocking battery life lies in the data. Core potential of battery data when utilising machine learning and data analytics methods can accurately determine, predict, and improve battery life. To achieve high battery efficiency and operational reliability, predictive intelligence and data analytics will play key roles as artificial intelligence becomes more disruptive in the battery technology space. The Battery Intelligence conference will bring thought leaders from industry and academia to discuss how organisations can use battery intelligence to improve battery life significantly and continuously.